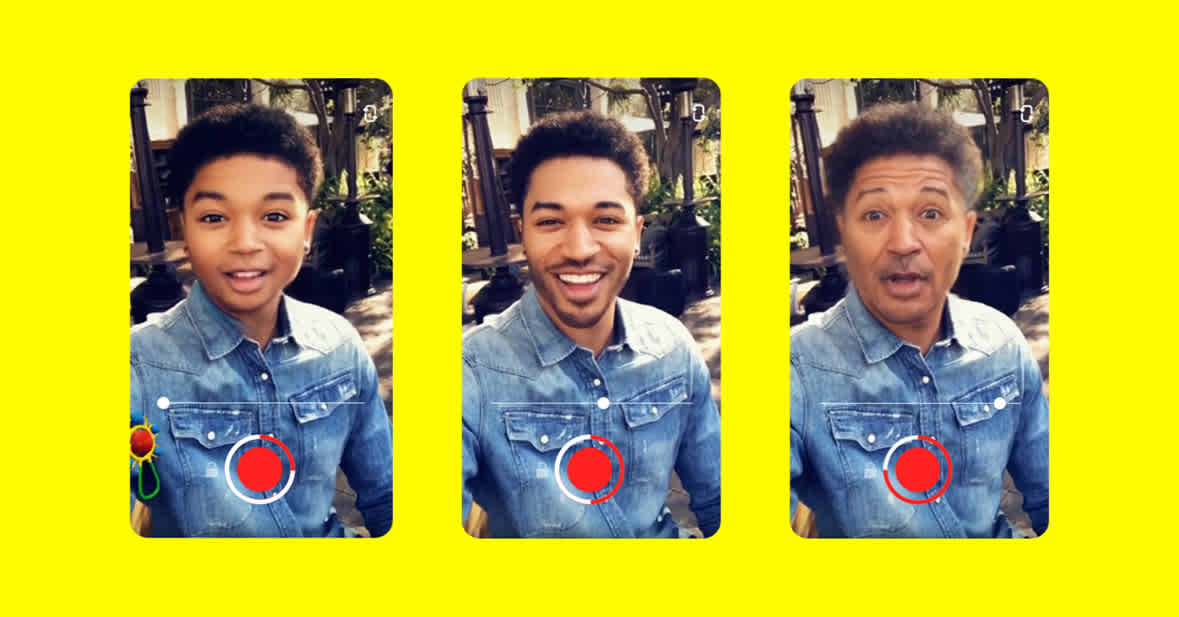
AI Lenses
Unified Recommendations
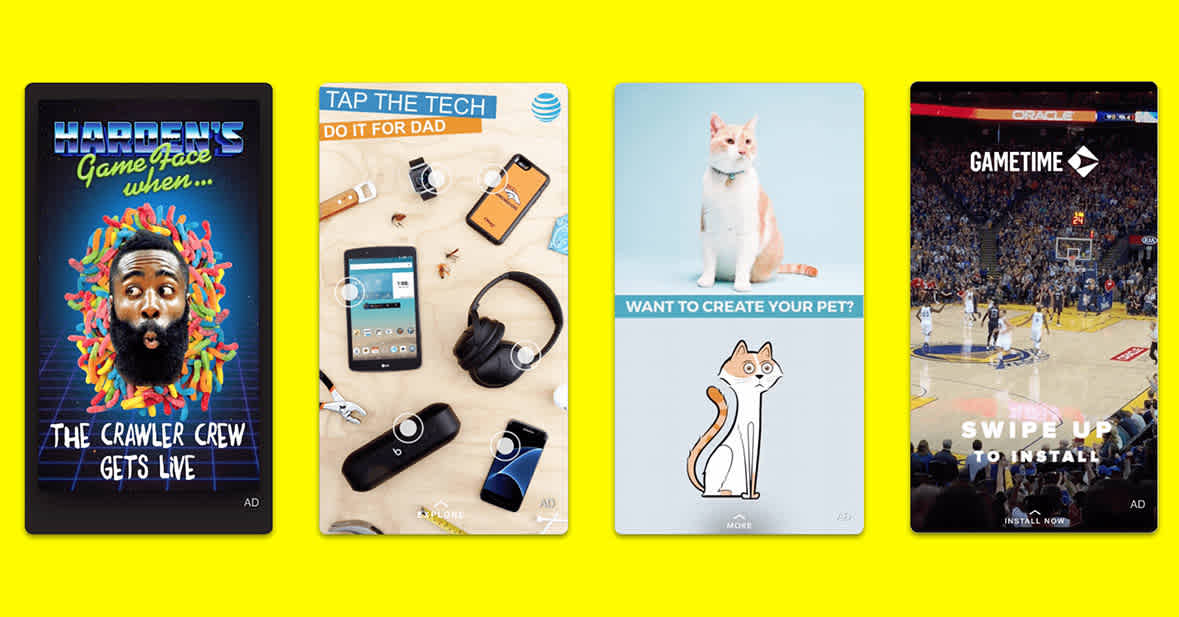
Dynamic Ads
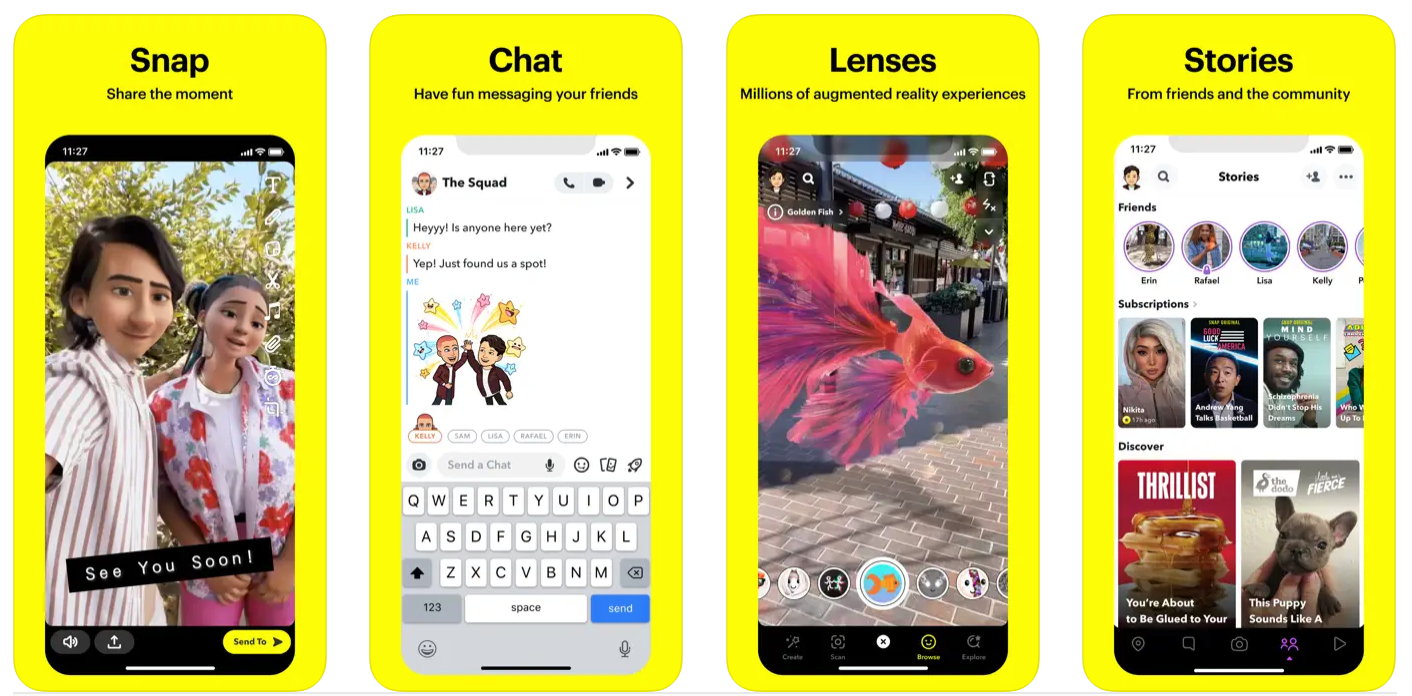
Machine Learning Engineering at Snap
As a Machine Learning Engineer at Snap you’ll drive Snapchat’s dynamic experience through the full lifecycle of advanced state-of-the-art models - from data preprocessing, feature engineering, and model training, to deployment and ongoing optimizations. You’ll leverage cutting-edge techniques, ranking algorithms for ad relevance, recommendation engines for personalized content and NLP for enhanced interactions - all while processing petabytes of data for over 850 million users.
Through both classic and deep learning models, you’ll create precise, responsive experiences that empower users to express themselves, connect and discover the world in real-time.
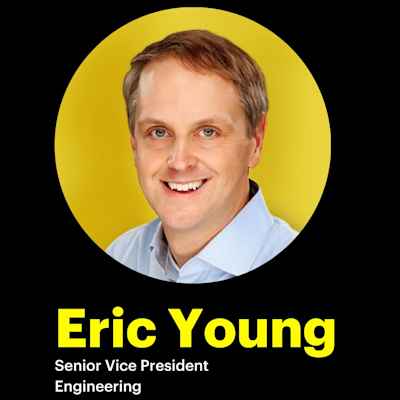
머신러닝 내의 여러 팀
Our machine learning engineers solve real world ML problems.
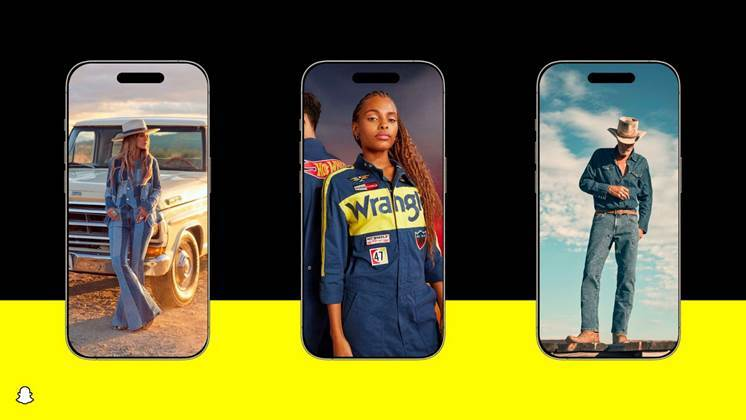
Monetization
As a Machine Learning Engineer on the Monetization team you’ll build and optimize the entire ad ecosystem. You’ll drive high-relevance and high-impact for not only advertisers and users but for all of Snap. From designing high-performance systems for real-time bidding or ad serving and auctions, personalizing light and heavy rankers, creating solutions for ad targeting and delivery, you’ll continue to ensure seamless integration of ads across the platform. You’ll train models on billions of examples, using multi-task learning, sequence modeling, and user x ad interaction modeling. Our models predict user demographics to improve audience targeting with graph neural networks and content, to understand how our work shapes the future of Snapchat’s ad platform.
What you’ll work on:
AI-driven advertising
New personalized ad products and experiences
Owner of Snap’s main revenue driver
Developing cutting edge ad products
Locations
Our RTO (Return to Office) policy is 4 times per week hiring in these office locations
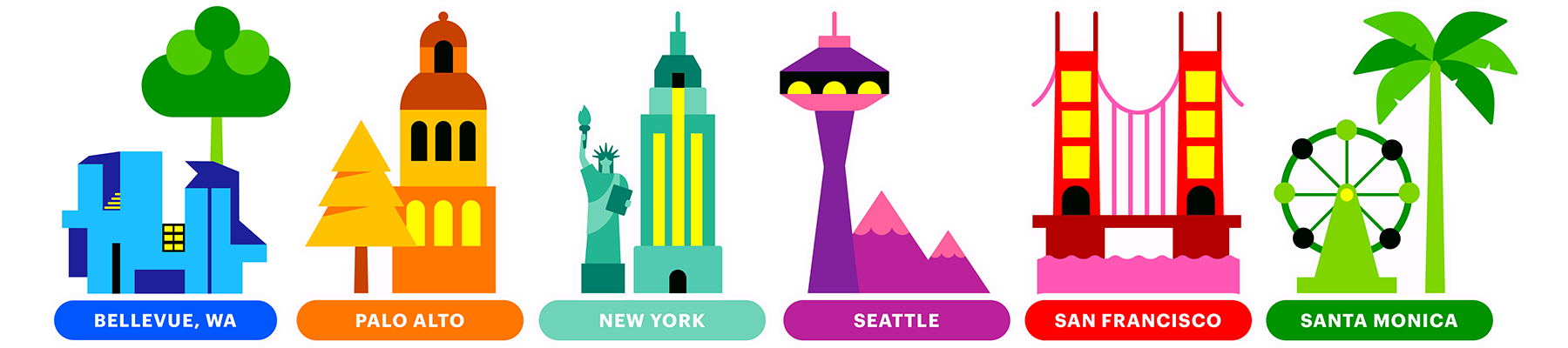
Snap에서의 ML
팀원들이 말하는 Snap 머신러닝에서 일하기
We're Hiring!
Our interview process covers engineering, foundational, and applied ML.
Coding
Expect to solve algorithmic problems that test your proficiency in data structures, algorithms, and problem-solving skills. Focus on your ability to write clean, efficient, and well-documented code.
ML Fundamentals
You’ll be assessed on ML theory and core machine learning models, concepts, techniques and applications. Be prepared to discuss supervised and unsupervised learning, recommendation systems, ranking, model evaluation metrics, and optimization techniques.
ML Applied Design
Evaluates your ability to design and apply machine learning solutions to real-world problems. You may be asked to walk through the end-to-end process of selecting models, feature engineering, and evaluating performance. At times this can test your ability to problem solve in an ambiguous environment.
ML System Design
The focus will be on designing scalable and robust ML systems that can handle large-scale data and production environments. Expect to discuss the infrastructure and trade offs in architecture, model deployment strategies and system monitoring.
Learn More
Ready to Build the Future?